semantic artificial inteligence
Semantic Artificial Intelligence based on Knowledge Graphs
The Future of Artificial Intelligence
The GNOSS project is part of the technological scenario of the Artificial Intelligence Program interpreted semantically or based on the exploitation by humans and machines of the inherent possibilities of linking data in a knowledge graph.
For more than 5 decades, DARPA (Defense Advanced Research Projects Agency) has led research and development that has enabled the advancement and application of rule-based Artificial Intelligence technologies and statistical learning. As DARPA (AI Next Campaign) highlights, the second wave of AI has come hand in hand with an explosion of interest in the AI subfield called machine learning, in which statistical and probabilistic methods are applied to massive data sets to create generalized representations that can be used in future data samples. One of the main approaches is deep learning with (artificial) neural networks that can be trained to perform various classification and prediction tasks when adequate historical data is available. However, therein lies your problem: the data collection, labeling and verification tasks with which to train these AI techniques can be costly and time consuming.
According to DARPA, next-generation AI will allow systems to add the ability to explain their results and equip them with reasoning with “common sense” insight.
The disambiguous and indubitable recognition of entities and their linking in a graph of significant knowledge, that is, that collects the rules that model the functioning of these entities in the world perceived by people, is a condition sine qua non for the construction of an AI endowed with "common sense".
In this new AI, some of the second wave techniques can and must converge that have proven particularly useful in specific tasks and that can be helpful when the volume of data sets makes it almost impossible for them to be managed by humans. In GNOSS projects, we apply neural models in information classification tasks that are part of the ETL (Extract Transform Load) processes towards the knowledge graph, such as the extraction of topics, the categorization of entities or the analysis of semantic similarity of texts ; and other techniques and algorithms on the graphs already built, in tasks such as the analysis of similarity of graphs or subgraphs or the recognition and disambiguation of entities (NERD) of GNOSS Sherlock.
Artificial Intelligence based on Knowledge Graphs
The brain stores our memories in a network of connected experiences forming a knowledge base. In GNOSS intelligent systems, this component is the knowledge graphs: a way of storing data that allows it to be given structure and context while enabling machines to learn and reason by exploiting their data and interrelations.
A knowledge graph is a particular type of graph that, according to the definition of Gartner (2018) “encodes information (“ knowledge ”) as data organized in a mesh or network (“ graph ”) of nodes and links (“ edges or arcs ”) instead of in row and column tables. Nodes contain data or its labels or metadata; the edges link nodes, representing the relationships between them. "
These relationships can be represented and made calculable using a special type of logic, First Order Predicate Logic; This kind of logic can represent these relationships as sequences of "triples", that is, node-edge-node, or "Velázquez-pintó-Las Meninas", which can accommodate, throughout the life cycle of the graph, diagrams of multiple and varied data without the need for redesign. Once encoded, the information can be retrieved or synthesized in response to any kind of query, including the most unexpected.
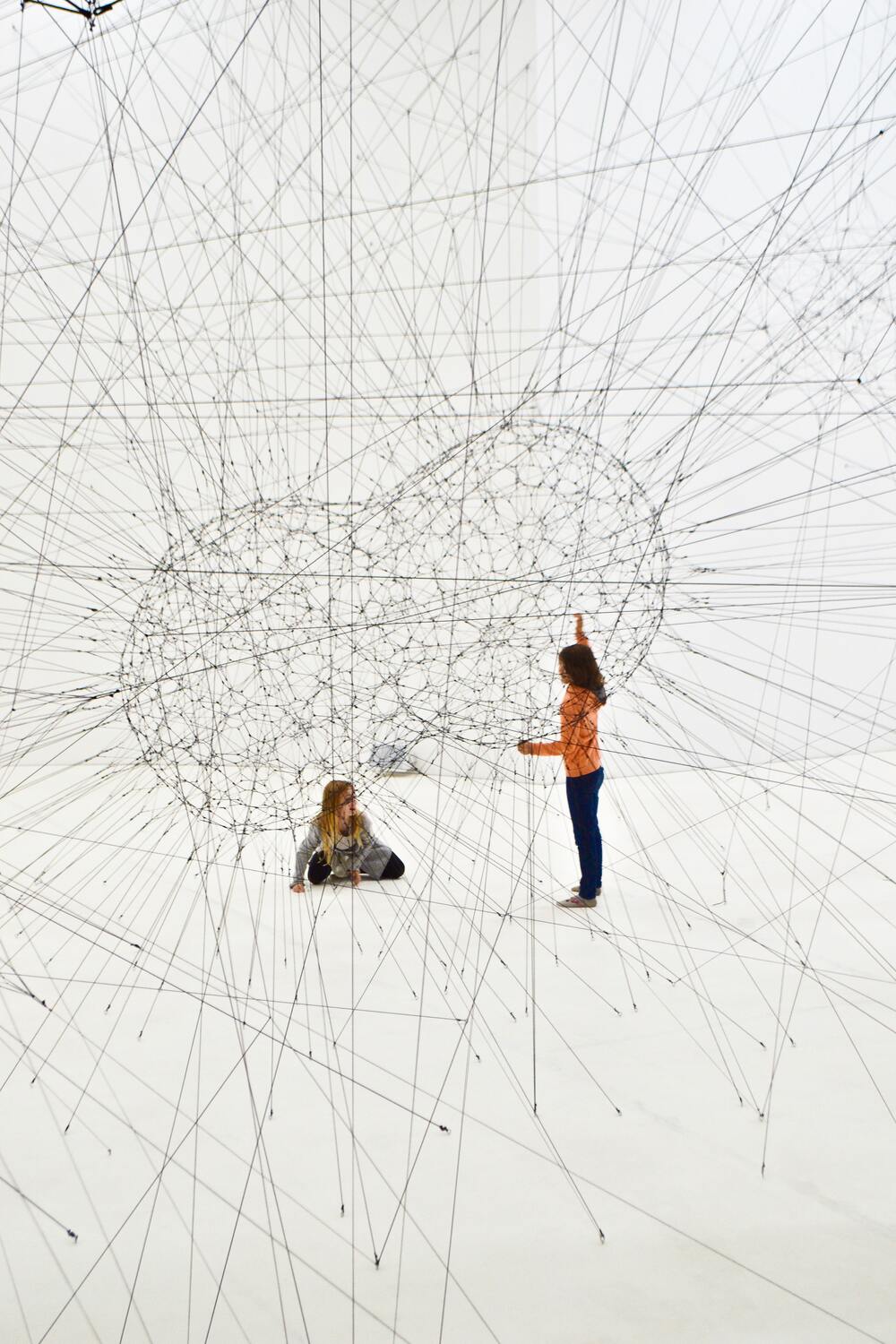
La creciente necesidad de integrar contenido heterogéneo y distribuido, de interpretarlo y dotarle de contexto ha llevado a los grafos de conocimiento a una situación prominente. Los casos del Knowledge Graph de Google o Microsoft Graph son ejemplos de su creciente utilidad y popularidad. Los grafos de conocimiento son el medio para enriquecer los datos con datos relacionados, pero que no están dentro de los sistemas de la organización.
Un grafo de conocimiento tiene cuatro grandes propiedades: unifica información heterogénea y distribuida, la hace interrogable por máquinas y personas, es expresivo y esta expresividad puede extenderse o ampliarse con facilidad.
Los grafos de conocimiento resultan una solución ideal para almacenar datos extraídos del análisis de fuentes no estructuradas, como documentos, utilizando el procesamiento en lenguaje natural (PNL). También son capaces de almacenar datos estructurados, incluidos los metadatos que, de manera implícita, proporcionan estructura y contexto a la información. En definitiva, los grafos de conocimiento permiten almacenar datos, dotarlos de estructura y contexto a la par que ofrecen sistemas de interrogación, recuperación de la información, descubrimiento de conocimiento y análisis que los hacen imprescindibles en un muy largo número de casos de uso.
Mediante el análisis basado en grafos de conocimiento, se pueden descubrir y explotar de manera automática las relaciones orgánicas y dinámicas entre activos digitales, fuentes de datos, procesos, personas e interacciones. Un aspecto clave a este respecto es la extracción de entidades. Las entidades (personas, organizaciones, lugares, eventos, etc.) pueden identificarse a través de técnicas de procesamiento del lenguaje natural antes de la ingesta de datos y posterior desambiguación. De este modo, los grafos de conocimiento acumulan silenciosamente "datos inteligentes", es decir, datos expresivos que los sistemas de Inteligencia Artificial apropiados pueden leer y "entender" fácilmente y cuya explotación ofrece resultados de alta calidad.
Deep knowledge of the intelligence functions of your company
GNOSS makes it possible for organizations to build their own knowledge graph, integrating in it the set of relevant information for the company. This knowledge graph facilitates the following functional exploitations: Knowledge discovery; Information enrichment and contextual information generation; and, User profiling and recommendation systems based on the explicit recognition of interests.
.jpg)
GNOSS makes it possible for organizations to build their own knowledge graph, integrating in it the set of information relevant to the company. This knowledge graph facilitates the following functional exploitations:
- Knowledge discovery
- Information interrogation, search and retrieval systems
- Search based on iterated constraints or natural reasoning: faceted search engines
- Searches based on iterated constraints or natural reasoning on maps
- Searches based on iterated restrictions or natural reasoning on ‘timelines’
- Interrogation based on graphic displays of indicators
- Navigation through entities
- Metadata management systems and labeling of company information
- Analysis systems based on interpreted data
- Business Analytics and Insights
- Learning Analytics
- Information interrogation, search and retrieval systems
- Information enrichment and contextual information generation
- User profiling and recommendation systems based on explicit recognition of interests
Business Impact of AI based on Knowledge Graphs
Organizations further on the frontier can expect to derive significant value from knowledge graphs in application areas that enable the construction of intelligence and learning functions, highlighting the following:
- - Collaboration, exchange and social learning
- - Investigation and audit
- - Analysis and reports
- - Integration and management of company content: interoperability and automation
- - Content management
- - Data reuse and collaboration between industries and companies
- - Customer relationship management
%20(1)%20(1).jpg)
Organizations further on the frontier can expect to derive significant value from knowledge graphs in application areas that enable the construction of intelligence and learning functions, highlighting the following areas:
- Collaboration, exchange and social learning: the consolidated data in a knowledge graph are interrelated and contextualized, which helps to discover and locate them through implicit and indirect connections. A knowledge graph can function as the "mind" of the company's collaboration and learning applications, such as:
- Social software in the workplace.
- Learning and training platforms
- Learning Analytics Systems
- Investigation and audit: the ability to capture and disambiguate entities that are assigned to individuals, things, organizations, places, events, manufacturing or distribution processes, etc. in the real world, it allows exploring the relationships between all of them to identify the origin of incidents, fraud, risks in supply chains, defects in manufacturing chains….
- Analysis and reports: once processed and interpreted, both structured and unstructured data, in a knowledge graph, these can be consulted, interrogated, integrated with third parties and viewed in an unmanaged, expressive and personal way, thus facilitating the extension of intelligence and analysis functions to the entire organization.
- Integration and management of company content: interoperability and automation. Autonomous reading and "understanding" of data by machines makes the integration and operability of data possible for the organization's set of applications.
- Content management:
- Organizational content management: intelligent integration, publication, distribution and notification, based on the profiling of the different audiences, of the organization's content.
- Communication, web content management and integration with external content.
- Data reuse and collaboration between industries and companies: data, in a knowledge graph, is linked conceptually or, if you prefer, semantically; this makes it easier for data and metadata to be combined and thus more easily shared and reusable.
- Customer relationship management: profiling and characterizing the customer experience. The CRM and the characterization of customers and their experience contribute to the design of business strategies that optimize profitability, operational efficiency, customer satisfaction and their loyalty through the implementation of customer-centric processes.
Smart Data for Predictive Analytics
The world and companies have been filled with data. But not all data tells stories. Big Data (Big Data) is concerned with the volume of data, the speed at which it is ingested, the variety of sources and its veracity, but the interpretation remains in the hands of the people who manipulate them. Interpreted Data (Smart Data) tell stories; They add to this the fact that the data know what it is and what others are related to and that it can be semantically interpreted by machines and systems.
.jpg)
The world and companies have been filled with data. But not all data tells stories. Big Data (Big Data) is concerned with the volume of data, the speed at which it is ingested, the variety of sources and its veracity, but the interpretation remains in the hands of the people who manipulate them. Interpreted Data (Smart Data) tell stories; They add to this the fact that the data know what they are and what others are related to and that they can be semantically interpreted by machines and systems.
Interpreted Data works like the atoms of a knowledge graph and associated analytical systems, and is the basis for building an Artificial Intelligence solution capable of answering the question "What can happen?" Predictive and trend and futures detection systems based on the analysis of interpreted big data represent a form of Artificial Intelligence that makes advanced analytics possible.
Companies that wish to consolidate their intelligence function are obliged to do predictive analysis based on interpreted data, consolidated in a knowledge graph that integrates and unifies company information with relevant information from abroad.
This set of techniques is framed within the field of Artificial Intelligence for analysis and definition of strategies, a central field of Business Intelligence. The objective is to identify strategies, practices, technologies and products that will create value for the business in the future.
Some of the most relevant use cases would be those that intend:
- demand prediction
- dynamic pricing
- predictive maintenance of equipment and machines
- the identification of fraud patterns and fraud detection